
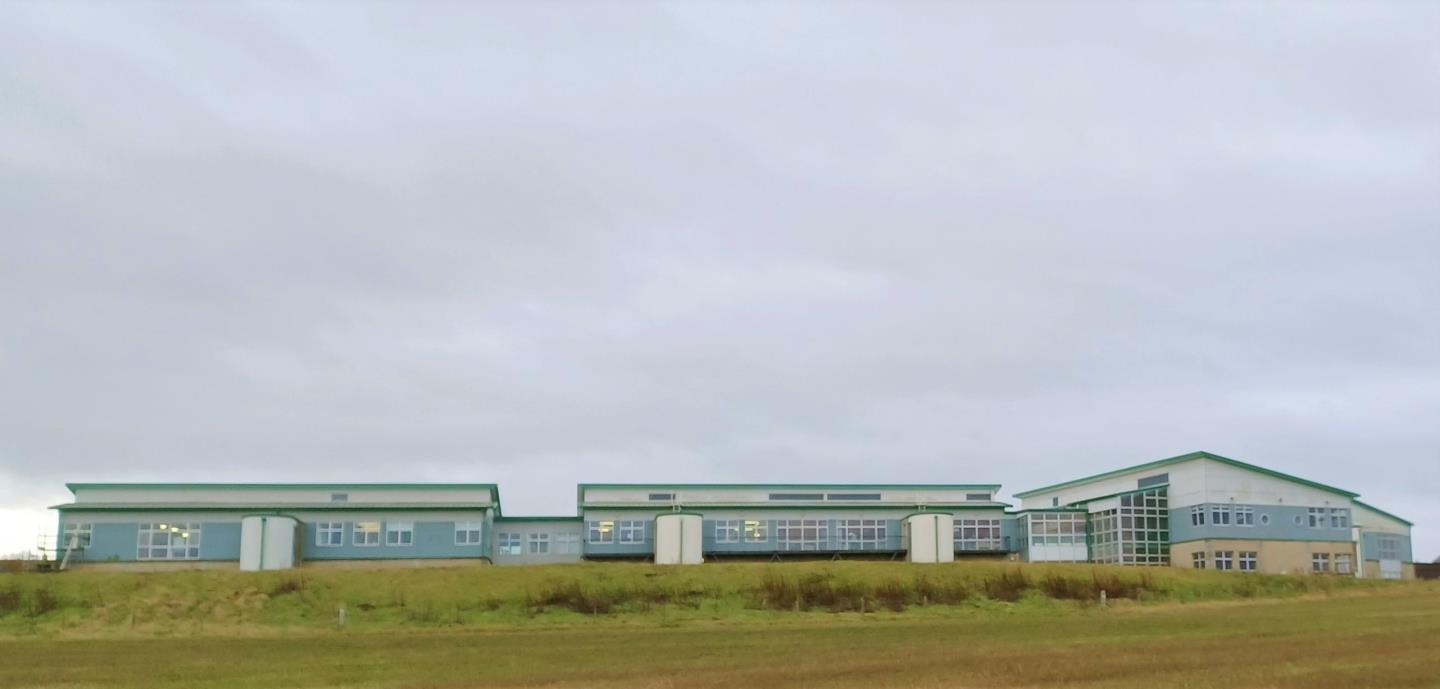
What happened? Well, as it turns out, it’s easy to find features that soak up variance in a training set, but those features might not be useful beyond the data they were trained on. But when I applied this model to the test data, the out-of-sample prediction accuracy actually DECREASED. When I added it to the model, it increased the in-sample prediction accuracy by several percentage points. I thought I’d found a significant new feature in the data. There were a few times where I encountered overfitting. More than I can possibly list, but here are some of Here are some of the major lessons. I learned a lot by tackling this challenge. In addition, although these features were not used to train the model per se, they were used later to adjust the predictions: Feature Grouped ticket prefixes (e.g., “SOTONO”), which presumably represent passengers’ destinationīayesian prediction of race/ethnicity using passenger surname
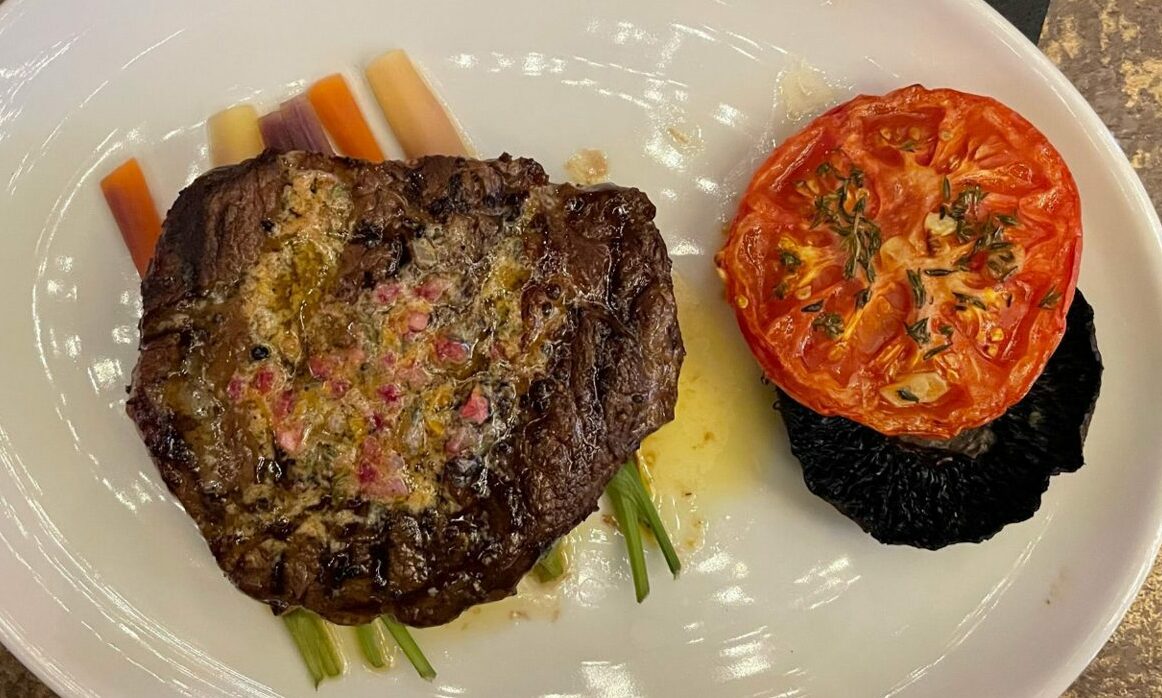
Honorifics (e.g., “Mr.” or “Master”), extracted from Name strings The following features were used in the model. These features involved transformations (e.g., binning) and “engineering” of existing variables (e.g., string extraction and manipulation). Your goal is to train a statistical model on traing data so that it can generate accurate predictions on the outcome of interest when applied to test data.įor this competition I created a model trained on 9 features of Titanic passengers. In this competition, the goal is to predict the survival of Titanic passengers whose fates are unknown, using what is known about some of the passengers who are known to have survived or perished. So I decided to try Kaggle’s Titanic competition. I want to learn new skills that will help me be a better data scientist, and machine learning seemes like a good place to start. I was trained as a behavioral scientist I know my way around a linear regression! But at the same time, I tend to use statistics to test formal hypotheses, and this seems (at least to an outsider like me) pretty different from machine learning.
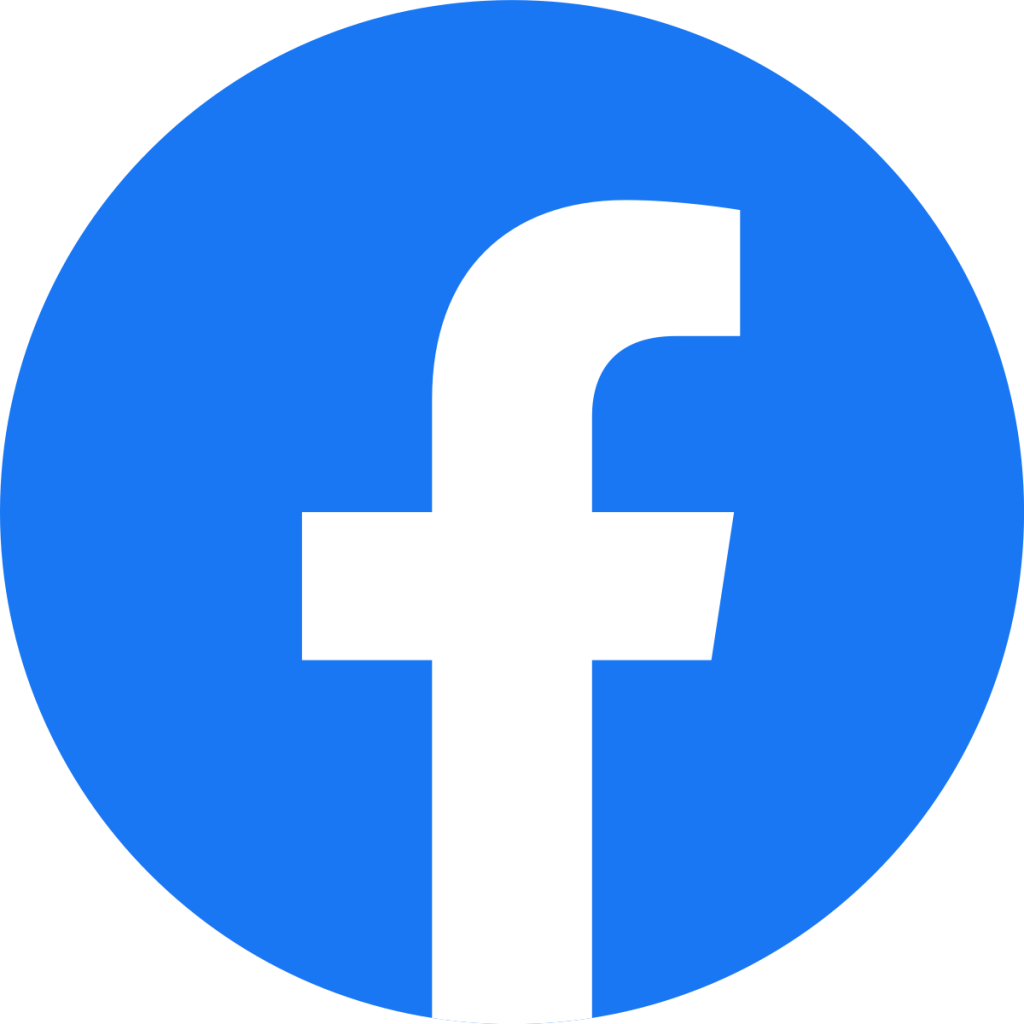
I’ve been meaning to “upskill” into the world of machine learning for some time.
